Local ML Model
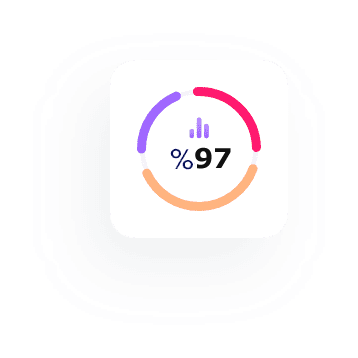
Offline-First Intelligence for Resilient Clinical Decision Support
In healthcare environments where consistent internet access is not guaranteed—such as mobile clinics, rural hospitals, disaster zones, and in-transit emergency care local AI deployment becomes essential.
ERTRIAGE’s on-device AI model architecture allows it to function as a fully autonomous decision-support tool, delivering real-time triage classifications without relying on cloud services or remote infrastructure.
On-device AI is an innovative approach allows us to run our artificial intelligence algorithms directly on core system, without needing to send data back and forth to remote real-time database for analysis. This approach to processing information where it’s collected—not in some distant data center—carries significant implications.
The local model is designed using TensorFlow Lite and optimized for ARM-based Android hardware, enabling low-latency AI inference directly on the device.
This empowers clinical and emergency teams to assess patients instantly, with no data transmission delays or dependency on external networks.
Key Functional Capabilities
- Full offline operation: No internet or server required for inference
- Response time: < 1 second for triage first-step decision or 180 sec for full triage mode
- Runs on: Android tablets, POI stations, EMS consoles, and rugged field devices
- Model Size: Lightweight neural network (<10MB) suitable for local memory footprint
Benefits of On-Device Deployment
- Reliability: Functions in connectivity-denied environments (e.g., disaster zones, ambulances, tactical deployments)
- Data Privacy: Patient information is never transmitted externally — all computation remains on the device
- Speed: Real-time feedback enables clinicians to act decisively under time pressure
- Independence: Reduces reliance on central IT infrastructure, ideal for standalone or temporary facilities
Clinical Scenario
A rural triage nurse receives a patient with symptoms of dehydration, tachycardia, and confusion. With no internet connectivity available, ERTRIAGE’s on-device model classifies the case as moderate-to-severe with high risk of sepsis. This classification supports the nurse’s decision to escalate care immediately and initiate IV fluid therapy without waiting for a physician or cloud-based decision system.
The local ML model is the foundation of ERTRIAGE’s resilience strategy — offering always-on, AI-driven triage support even in the most challenging and disconnected clinical environments.
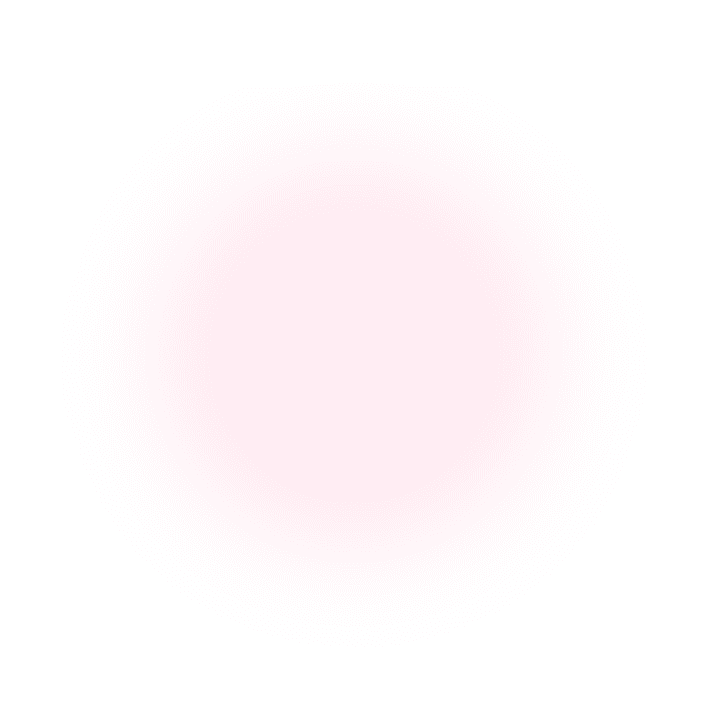
ERTRIAGE AI system
Final Thoughts
ERTRIAGE is more than a product. It’s a platform, a tool, and a partner to the clinicians who serve on the frontlines of care. By combining AI, modern UX, secure engineering, and clinical wisdom, we’ve created a system that helps emergency teams focus on what matters most: the patient.
We believe this is the future of emergency triage—and we’re proud to have pioneered it.
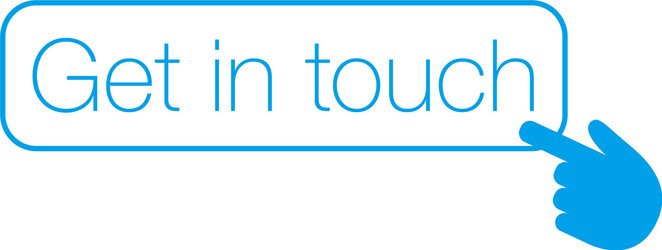